Examine Amazon's Predictive Analytics for Product Recommendations & Revenue Growth
Amazon, the e-commerce giant, has revolutionised retail with its sophisticated use of predictive analytics. Central to its success are algorithms that analyse vast amounts of data to offer personalised product recommendations to customers. This blog delves into how Amazon utilises predictive analytics for product recommendations and how this strategy drives revenue growth.
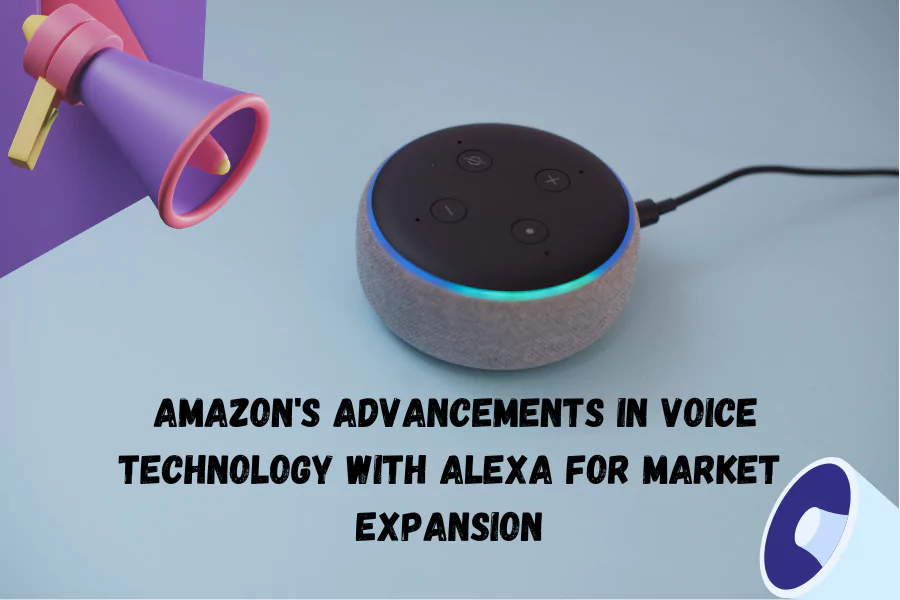
The Power of Predictive Analytics at Amazon
Amazon's success in predictive analytics lies in its ability to harness data from various sources:
- User Behavior Analysis: Amazon collects data on user browsing history, purchase patterns, search queries, and even mouse movements. This data helps build detailed customer profiles and understand individual preferences.
- Product Catalogue Data: Amazon analyses product attributes, customer reviews, ratings, and sales data to identify correlations and trends. This helps in understanding which products are popular and which attributes contribute to customer satisfaction.
- External Data Sources: Amazon integrates external data sources such as weather forecasts, social media trends, and economic indicators. This broader data context enriches predictive models, allowing for more accurate predictions.
Product Recommendations Engine
Amazon's product recommendations engine is a cornerstone of its personalised shopping experience:
- Collaborative Filtering: Amazon employs collaborative filtering algorithms that analyse user behaviour and similarities with other users. This approach recommends products based on what similar users have viewed, purchased, or liked.
- Content-Based Filtering: Amazon also uses content-based filtering to recommend products based on attributes and characteristics of items that a user has shown interest in. This method considers product features, descriptions, and categories.
- Machine Learning Algorithms: Amazon continuously refines its recommendation engine using machine learning algorithms. These algorithms adapt to new data in real-time, improving the accuracy and relevance of recommendations over time.
Driving Revenue Growth
Amazon's use of predictive analytics for product recommendations directly contributes to revenue growth:
- Increased Sales: Personalised recommendations lead to higher conversion rates as customers are more likely to purchase products tailored to their interests and needs. This boosts average order value and overall sales revenue.
- Enhanced Customer Experience: By offering relevant suggestions, Amazon enhances the shopping experience, making it more convenient and enjoyable for customers. This positive experience encourages repeat purchases and builds customer loyalty.
- Cross-Selling and Up-Selling: Predictive analytics enables Amazon to effectively cross-sell related products and up-sell higher-value items. For example, suggesting complementary items or premium versions of products based on customer preferences.
- Reduced Cart Abandonment: Personalised recommendations help mitigate cart abandonment by guiding customers towards products they are more likely to buy, thereby optimising the purchasing journey.
Case Studies of Success
Amazon's predictive analytics for product recommendations have been instrumental in its growth:
- "Customers who bought this item also bought...": This feature prominently displays related products based on collaborative filtering, encouraging additional purchases.
- Personalised Homepage: Amazon customises the homepage for each user based on their browsing and purchase history, showcasing products and deals tailored to individual preferences.
- Prime Day Recommendations: During Prime Day and other sales events, Amazon uses predictive analytics to highlight deals personalised to each customer’s interests, driving significant sales volumes.
Amazon's predictive analytics for product recommendations exemplify the transformative impact of data-driven decision-making in e-commerce. By leveraging vast amounts of data, sophisticated algorithms, and machine learning, Amazon enhances customer engagement, drives revenue growth, and sets industry standards for personalised shopping experiences. As Amazon continues to innovate in predictive analytics, its ability to anticipate customer needs and deliver relevant recommendations will likely remain a key competitive advantage in the ever-evolving retail landscape.
Active Events
Navigating the World of SERP Features: Tips, Tricks, and Strategies
Date: December 4, 2024 | 7:00 PM(IST)
7:00 PM(IST) - 8:10 PM(IST)
2811 people have registered
Best Tips to Create a Job-Ready Data Science Portfolio
Date: October 31, 2024 | 7:00 PM(IST)
7:00 PM(IST) - 8:10 PM(IST)
2811 people have registered
Bootcamps
Digital Marketing Bootcamp
- Duration:4 Months
- Start Date:Dec 8, 2024
Data Science Bootcamp
- Duration:4 Months
- Start Date:Dec 8, 2024