Education and Student Performance
Data analysis plays a crucial role in understanding and improving education and student performance. Here's a breakdown of how data can be used in this context:
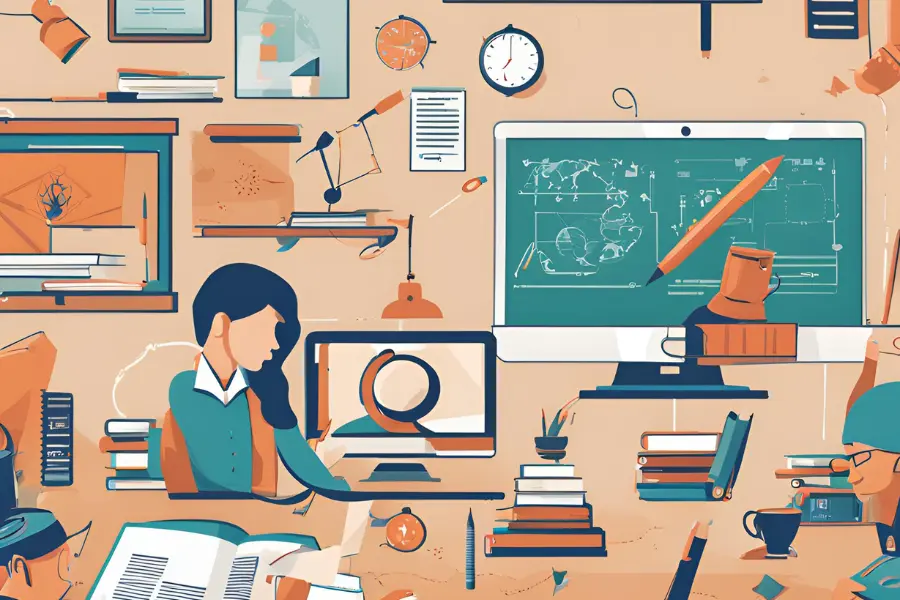
Monitoring and Tracking Progress
- Attendance Records: Tracking student attendance helps identify patterns and potential issues that could affect performance.
- Grades and Test Scores: Analyzing these can highlight strengths and weaknesses in student performance, guiding targeted interventions.
- Behavioral Data: Monitoring behavior incidents can provide insights into underlying issues affecting learning.
Personalized Learning
- Learning Analytics: Data from digital learning platforms can be used to tailor educational content to individual students' needs.
- Adaptive Learning Technologies: These systems use data to adapt the learning experience in real-time, providing customized support.
Identifying At-Risk Students
- Predictive Analytics: Using historical data to identify students who are at risk of falling behind or dropping out.
- Early Warning Systems: Implementing systems that alert educators to students who may need additional support.
Improving Teaching Strategies
- Feedback from Assessments: Analyzing test results to understand which teaching methods are most effective.
- Professional Development: Data can identify areas where teachers may need additional training or resources.
Resource Allocation
- Financial Data: Analyzing budgets to ensure that resources are being used efficiently to support student learning.
- Infrastructure Utilization: Understanding how physical and digital resources are being used to optimize their deployment.
Curriculum Development
- Curriculum Effectiveness: Evaluating the impact of different curricular approaches on student outcomes.
- Alignment with Standards: Ensuring that the curriculum meets educational standards and benchmarks.
Engagement and Satisfaction
- Surveys and Feedback: Collecting data from students, parents, and teachers to gauge satisfaction and engagement levels.
- Extracurricular Activities: Analyzing participation in and the impact of extracurricular activities on overall student development.
Equity and Inclusion
- Demographic Data: Ensuring that all student groups have equitable access to educational opportunities.
- Achievement Gaps: Identifying and addressing disparities in performance among different student groups.
Policy Making and Planning
- Evidence-Based Decision Making: Using data to inform educational policies and strategic planning.
- Longitudinal Studies: Conducting long-term studies to understand trends and the impact of educational initiatives over time.
Examples of Data Analysis in Education
- Descriptive Statistics: Summarizing data to understand overall trends (e.g., average test scores, graduation rates).
- Inferential Statistics: Making predictions or inferences about a larger population based on sample data.
- Machine Learning: Applying algorithms to identify patterns and make predictions about student performance.
- Data Visualization: Creating charts and graphs to make data more accessible and actionable for educators and administrators.
Tools and Technologies
- Learning Management Systems (LMS): Platforms like Blackboard, Canvas, and Moodle that collect and manage educational data.
- Student Information Systems (SIS): Systems like PowerSchool and Infinite Campus that manage student records.
- Data Analytics Platforms: Tools like Tableau, Power BI, and R for analyzing and visualizing educational data.
- AI and Machine Learning: Technologies that provide deeper insights and predictive analytics.
Using data effectively in education can lead to more informed decision-making, better support for students and teachers, and overall improved educational outcomes.
Active Events
3 Must Have Projects On your CV to Get into Data Analysis
Date: Feburary 25, 2025 | 7:00 PM(IST)
7:00 PM(IST) - 8:10 PM(IST)
2753 people registered
Transition from Non-Data Science to Data Science Roles
Date: Feburary 27, 2025 | 7:00 PM (IST)
7:00 PM (IST) - 8:10 PM (IST)
2753 people have registered
Bootcamps
BestSeller
Data Analyst Bootcamp
- Duration:8 weeks
- Start Date:October 5, 2024
BestSeller
Digital Marketing Bootcamp
- Duration:8 weeks
- Start Date:October 5, 2024