Examine the Impact of Deep Learning on Google’s Search Ranking Algorithms
Deep learning has profoundly influenced Google's search ranking algorithms, enhancing their ability to deliver relevant, high-quality search results. Here’s an in-depth look at how deep learning impacts Google’s search ranking:
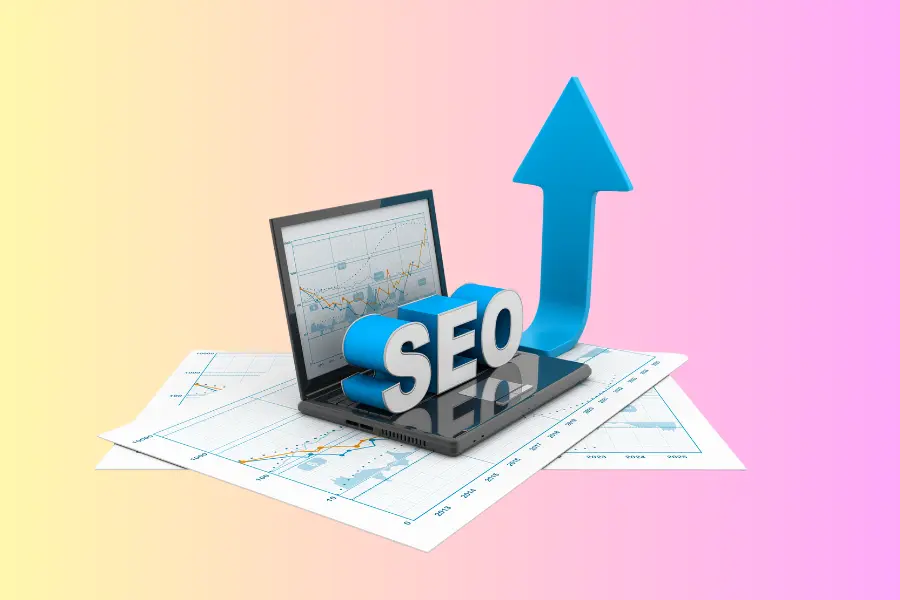
1. Introduction to Deep Learning
Deep learning, a subset of machine learning, involves neural networks with multiple layers that can learn and make decisions from vast amounts of data. These networks, especially deep neural networks, excel in recognizing patterns and extracting features from complex datasets.
2. Deep Learning Models in Google Search
Google has integrated several deep learning models into its search ranking algorithms:
- RankBrain: Introduced in 2015, RankBrain is a machine learning-based component of Google's search algorithm. It helps process and understand search queries by analysing the context and intent behind them, improving the relevance of search results.
- Neural Matching: This deep learning system, rolled out in 2018, helps Google understand how words in a query relate to concepts in the indexed content. It enhances the ability to match search queries with relevant pages that may not contain the exact keywords.
- BERT (Bidirectional Encoder Representations from Transformers): Implemented in 2019, BERT is a deep learning model designed to better understand the context of words in search queries. It processes words in relation to all other words in a sentence, allowing Google to grasp the nuances and intricacies of natural language.
3. Impact on Search Ranking Algorithms
Deep learning models have significantly improved Google’s search ranking algorithms in several ways:
- Enhanced Query Understanding: Deep learning allows Google to better understand the intent behind search queries, especially complex or conversational ones. This leads to more accurate and contextually relevant search results.
- Improved Relevance and Quality: By analysing content more deeply and understanding the relationships between words and concepts, deep learning models help Google rank pages based on their true relevance and quality, rather than just keyword matching.
- Semantic Search: Deep learning enhances semantic search capabilities, enabling Google to understand the meaning behind queries and content. This improves the search experience by delivering results that align more closely with user intent.
- Contextual Awareness: Models like BERT enhance contextual awareness in search queries. For example, understanding the difference between "bank" as a financial institution and "bank" as a riverbank based on the context provided by surrounding words.
4. Examples of Deep Learning Impact
Deep learning has led to several impactful changes:
- Voice Search and Conversational Queries: Deep learning improves the accuracy of voice search by understanding conversational language. This leads to more precise results for spoken queries.
- Featured Snippets and Rich Results: Deep learning helps Google extract and display relevant information in featured snippets and rich results, providing users with quick answers to their queries.
- Handling Ambiguity and Polysemy: Deep learning models are better equipped to handle ambiguous queries and words with multiple meanings, delivering results that are contextually appropriate.
5. Challenges and Considerations
While deep learning has significantly enhanced Google’s search capabilities, it also presents challenges:
- Computational Resources: Deep learning models require substantial computational power and resources for training and inference.
- Transparency and Interpretability: Deep learning models, especially deep neural networks, can be complex and opaque, making it difficult to understand how decisions are made. Google must balance performance with transparency and interpretability.
- Bias and Fairness: Ensuring that deep learning models do not propagate biases present in training data is crucial. Google must continuously monitor and mitigate potential biases to maintain fairness in search results.
6. Future Directions
Continued advancements in deep learning are expected:
- Continued Integration of Advanced Models: Google is likely to integrate more advanced deep learning models, such as those based on transformer architectures, to further enhance search capabilities.
- Real-Time Updates and Adaptation: Deep learning models can be updated and adapted in real-time, allowing Google to respond quickly to emerging trends and changes in user behaviour.
- Personalization: Deep learning can further improve personalised search experiences by understanding individual user preferences and delivering tailored results.
Deep learning has revolutionised Google’s search ranking algorithms, enabling more accurate, relevant, and contextually aware search results. By leveraging advanced neural networks and models like RankBrain, Neural Matching, and BERT, Google continues to enhance the search experience for users worldwide. As deep learning technology evolves, we can expect even greater advancements in how search engines understand and respond to user queries.
Active Events
Data Scientist Challenges One Should Avoid
Date: Feburary 25, 2025 | 7:00 PM (IST)
7:00 PM (IST) - 8:10 PM (IST)
2753 people have registered
Your Data Science Career Game-Changing in 2024: Explore Trends and Opportunities
Date: Feburary 28, 2025 | 7:00 PM (IST)
7:00 PM (IST) - 8:10 PM (IST)
2811 people have registered
Bootcamps
Data Science Bootcamp
- Duration:8 weeks
- Start Date:October 5, 2024
Full Stack Software Development Bootcamp
- Duration:8 weeks
- Start Date:October 5, 2024