Navigate
Leveraging Quantum Computing for Next-Generation Data Science Solutions
- Quantum Machine Learning: Revolutionising Predictive Analytics
- Quantum Data Encryption: Ensuring Unparalleled Security in Data Science
- Quantum Data Compression: Optimising Storage and Transmission Efficiency
- How Quantum Algorithms Improve Fraud Detection in Social Network Transactions?
- How Quantum Algorithms Revolutionize Financial Portfolio Optimization for Risk Management?
- Explore the Impact of Google's quantum supremacy on data science applications
Unlocking Hidden Insights: A unique Approach to Data Science Excellence
Google’s Data Science Approach to Staying Ahead in Search and Advertising
How Amazon Uses Data Science to Drive E-commerce Innovation
How Quantum Algorithms Revolutionise Financial Portfolio Optimization for Risk Management?
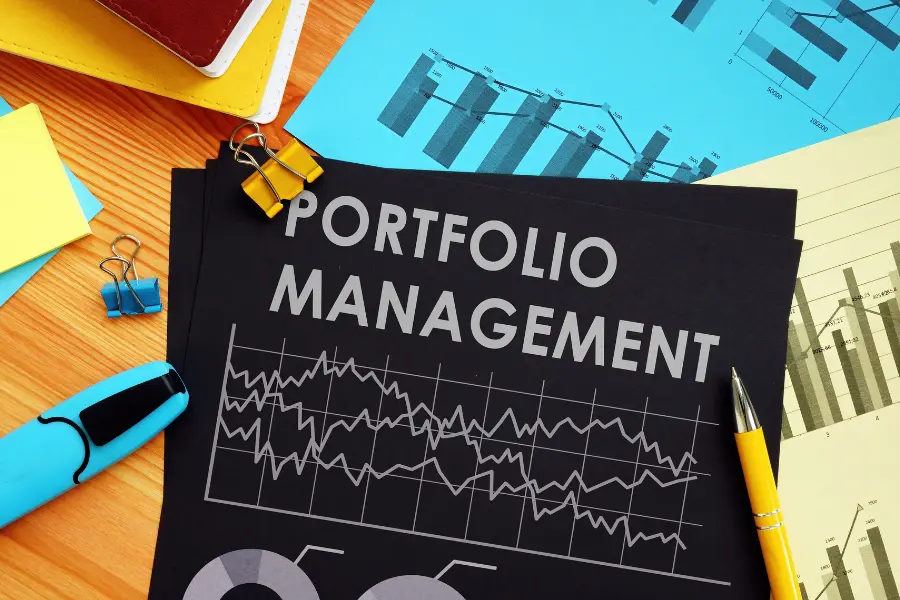
Quantum algorithms have the potential to revolutionise financial portfolio optimization for risk management by leveraging the unique computational advantages offered by quantum computing. Here’s an exploration of how quantum algorithms could transform portfolio optimization:
Quantum Algorithms and Financial Portfolio Optimization
1. Traditional Portfolio Optimization Challenges
Traditional portfolio optimization involves selecting a combination of assets that maximises returns while minimising risk, considering factors such as asset correlations, volatility, and return expectations. Classical approaches often face challenges in handling large datasets and complex optimization models efficiently.
2. Quantum Computing Basics
Quantum computing operates on principles such as superposition and entanglement, allowing quantum algorithms to explore multiple solutions simultaneously. This capability potentially enables quantum computers to solve complex optimization problems faster than classical computers.
3. Potential Benefits of Quantum Algorithms
Speed and Efficiency: Quantum algorithms can explore a vast number of potential portfolio combinations and optimization strategies simultaneously, leading to faster computation of optimal portfolios compared to classical methods.
Complexity Handling: Quantum computers excel in handling complex, high-dimensional datasets and optimization models that involve nonlinear relationships and constraints, which are critical in portfolio optimization for risk management.
Global Optimization: Quantum algorithms could enable the discovery of globally optimal portfolio solutions that classical algorithms might miss due to computational limitations.
4. Application Scenarios
Efficient Frontier Analysis: Quantum algorithms can efficiently compute the efficient frontier, which represents the set of optimal portfolios that offer the highest expected return for a given level of risk or the lowest risk for a given level of return.
Risk Assessment and Mitigation: Quantum computing could enhance the accuracy and speed of risk assessment models by considering a broader range of risk factors and scenarios in real-time.
Diversification Strategies: Quantum algorithms can optimize asset allocation strategies that maximize diversification benefits while minimizing portfolio risk, considering correlations and volatility across multiple asset classes.
5. Challenges and Considerations
Hardware Limitations: Current quantum computers have limited qubits and coherence times, restricting the complexity and size of optimization problems they can effectively solve. Scaling quantum algorithms for practical portfolio optimization remains a challenge.
Algorithm Development: Designing quantum algorithms for portfolio optimization requires expertise in quantum computing and finance to translate classical optimization models into quantum-compatible formulations.
Integration with Classical Systems: Hybrid quantum-classical approaches may be necessary to leverage the strengths of both quantum and classical computing for practical portfolio optimization solutions.
6. Future Directions
Research and Development: Continued research aims to develop and refine quantum algorithms specifically tailored for financial portfolio optimization, focusing on scalability, accuracy, and applicability in real-world financial markets.
Quantum Software Ecosystem: The development of quantum software frameworks and tools that facilitate the implementation and deployment of quantum algorithms for portfolio optimization is crucial for adoption.
Quantum algorithms have the potential to revolutionize financial portfolio optimization by offering faster, more efficient solutions to complex risk management challenges. While current quantum computing technology is still evolving, ongoing research and advancements are expected to pave the way for transformative applications in optimizing investment portfolios and managing financial risks more effectively.