How Quantum Algorithms Improve Fraud Detection in Social Network Transactions
Quantum Algorithms and Fraud Detection in Social Networks
Quantum algorithms hold promise in enhancing fraud detection in social network transactions by leveraging quantum computing's unique capabilities. Here's an exploration of how quantum algorithms could potentially improve fraud detection:
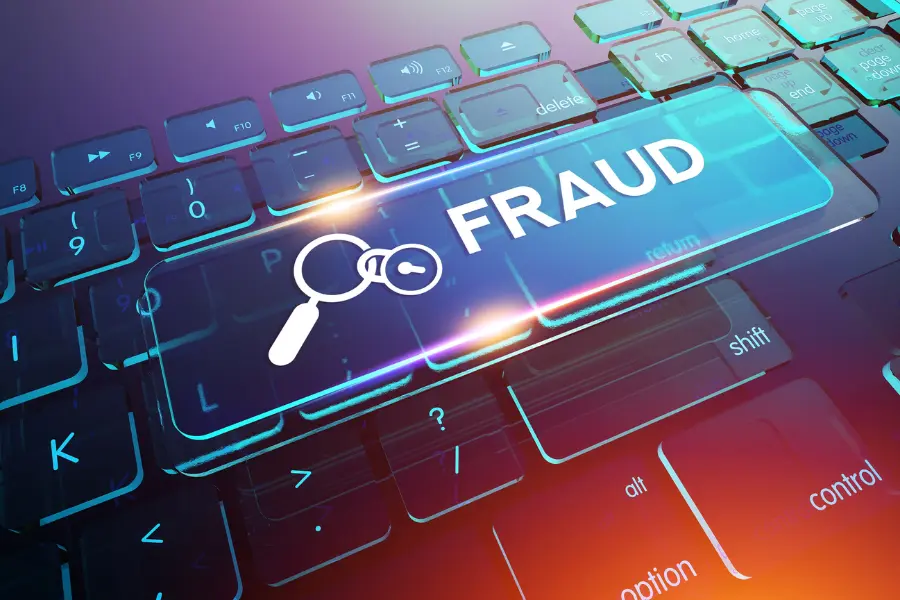
1. Quantum Computing Basics
Quantum computing utilises quantum bits or qubits, which can exist in multiple states simultaneously due to principles like superposition and entanglement. This parallel processing capability allows quantum computers to solve certain complex problems much faster than classical computers.
2. Fraud Detection Challenges
Fraud detection in social network transactions involves analysing large volumes of transactional data to identify patterns indicative of fraudulent activities. Traditional methods often struggle with processing vast datasets in real-time and detecting subtle, evolving fraud patterns.
3. Potential Benefits of Quantum Algorithms
Quantum algorithms offer several potential advantages for enhancing fraud detection:
- Parallel Processing: Quantum computers can simultaneously explore multiple paths and possibilities, enabling faster analysis of large datasets.
- Optimization and Pattern Recognition: Quantum algorithms could improve the accuracy and efficiency of identifying fraudulent patterns that classical algorithms might miss.
- Complexity Handling: Quantum algorithms excel in handling complex, nonlinear relationships and dependencies within data, crucial for detecting coordinated fraud rings.
4. Application Scenarios
- Graph Analytics: Quantum algorithms can analyse the complex networks of relationships and transactions in social networks more efficiently than classical graph-based methods. This capability is crucial for detecting coordinated fraud rings or anomalous behaviour patterns.
- Feature Extraction and Analysis: Quantum machine learning algorithms could enhance feature extraction from transactional data, identifying subtle indicators of fraud that classical methods might overlook.
- Real-Time Decision Making: Quantum algorithms, when integrated with real-time data streams from social networks, could enable quicker decision-making in response to potential fraud incidents, reducing financial losses and improving security.
5. Challenges and Considerations
- Hardware Limitations: Current quantum computers are in early stages of development and have limited qubits and coherence times. Scaling quantum algorithms for practical fraud detection applications remains a significant challenge.
- Algorithm Development: Designing and optimising quantum algorithms for specific fraud detection tasks requires specialised expertise and may involve adapting classical algorithms to quantum computing paradigms.
- Security and Privacy: Quantum computing also poses potential risks to security protocols and encryption standards currently used to protect transactional data. Addressing these challenges is crucial for safe implementation.
6. Future Directions
- Research and Development: Ongoing research aims to develop quantum algorithms tailored for fraud detection, focusing on scalability, accuracy, and real-time applicability.
- Hybrid Approaches: Hybrid quantum-classical algorithms that leverage the strengths of both quantum and classical computing could provide more practical solutions for fraud detection in the near term.
Quantum algorithms hold promise for revolutionising fraud detection in social network transactions by leveraging quantum computing's parallel processing capabilities and ability to handle complex, interconnected datasets. While current quantum computing technology is still evolving, ongoing research and advancements are expected to pave the way for more effective and efficient fraud detection solutions in the future.
Active Events
Best Tips to Create a Job-Ready Data Science Portfolio
Date: Feburary 26, 2025 | 7:00 PM(IST)
7:00 PM(IST) - 8:10 PM(IST)
2811 people have registered
Unlock Your Career Potential: The 2024 Full Stack Development Success Formula
Date: Feburary 28, 2025 | 7:00 PM(IST)
7:00 PM(IST) - 8:10 PM(IST)
2749 people have registered
Bootcamps
Data Science Bootcamp
- Duration:8 weeks
- Start Date:October 5, 2024
Full Stack Software Development Bootcamp
- Duration:8 weeks
- Start Date:October 5, 2024