Quantum Machine Learning: Revolutionising Predictive Analytics
Quantum Machine Learning (QML) represents a dynamic shift in predictive analysis to redefine how we use data and make estimations. It merges principles from quantum mechanics with techniques from machine learning. It explores how quantum computing can enhance and optimise various aspects of traditional machine learning algorithms and processes. Quantum Machine Learning tries to discover the unique properties of quantum computing, such as positioning and collaboration to improve the efficiency and effectiveness of machine learning tasks. This includes data classification, pattern recognition, optimization problems, and predictive analytics.
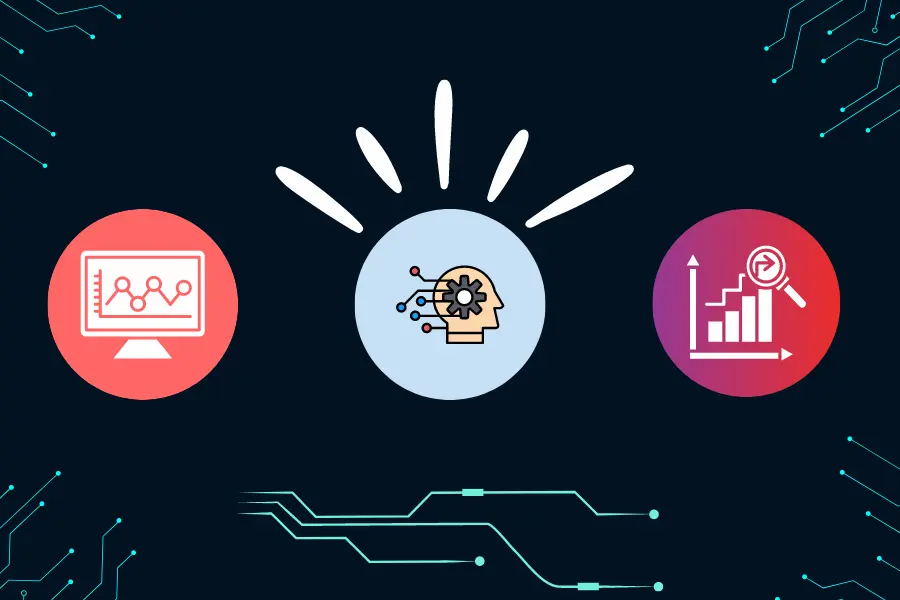
The Power of Quantum Computing
Quantum computing stands at the age of revolutionising information processing, promising to deliver computational power that overlooks even the most sophisticated classical computers. This emerging field leverages the principles of quantum mechanics to perform complex calculations at unmatched speeds, potentially transforming areas such as artificial intelligence, cybersecurity, and machine learning. As we edge closer to integrating quantum computing into practical applications, understanding its fundamentals, capabilities, and implications becomes crucial for anyone looking to navigate the future of technology.
Quantum machine learning integrates quantum computing principles with classical machine learning algorithms to potentially enhance optimization processes. Quantum computers, leveraging qubits (or quantum bit- is the basic unit of information in quantum computing) in superposition, can explore exponentially larger solution spaces, offering significant speedups in tasks like optimization and classification. Quantum Annealing, for example, uses quantum fluctuations to find the lowest energy state of an objective function, while Variational Quantum Algorithms optimise objective functions through imperatively updated quantum circuits. Quantum Support Vector Machines (QSVM) utilise quantum principles to improve classification performance, particularly in high-dimensional feature spaces. These advancements in quantum machine learning promise faster processing times and open up new possibilities for handling complex data sets and models.
Quantum Algorithms for Predictive Analytics
Quantum Machine Learning (QML) opens the way for a new era in predictive analytics by leveraging quantum algorithms that surpass the limitations of traditional machine learning methods. Algorithms like the Quantum Support Vector Machine (QSVM) and Quantum Neural Network (QNN) capitalise on the distinct properties of qubits to process vast and complex datasets with enhanced efficiency. This empowers QML to deliver predictions with greater accuracy and get deeper insights by simultaneously exploring multiple solutions.
By harnessing the basic parallelism of quantum computing, QML identifies subtle patterns that may avoid classical algorithms, unlocking new avenues for predictive analysis and transforming our understanding of complex data landscapes. This pattern shift in predictive analytics promises to revolutionise various domains, from finance and healthcare to e-commerce, by enabling more precise predictions and uncovering previously out-of-reach insights. Quantum algorithms are all set to revolutionise computing by transforming various fields, including cryptography, optimization, and material science, by tackling tasks that are currently beyond the reach of classical computers.
Applications Across Industries
Quantum Machine Learning (QML) has the potential to revolutionise many industries with its transformative applications.
In Finance: QML algorithms can sift through massive financial datasets to uncover intricate patterns and trends, leading to more accurate risk assessments and better investment predictions.
In Healthcare: QML can analyse patient data to predict disease outcomes and spot early warning signs, helping to create personalised treatment plans.
In E-commerce: QML improves recommendation systems by examining user behaviour data to offer customised product suggestions, thereby boosting customer engagement and satisfaction. The far-reaching implications of QML span various sectors, promising advancements in financial analysis, healthcare personalization, and e-commerce customization.
Quantum Machine Learning (QML) is also making progress in the field of cybersecurity. By leveraging quantum algorithms, QML can enhance encryption methods, making data more secure against cyber threats. Traditional encryption methods are becoming increasingly vulnerable to sophisticated hacking techniques, but quantum encryption, powered by QML, offers a level of security that is currently unmatched. Furthermore, QML can be used to detect and respond to security breaches in real time by analysing network traffic patterns and identifying anomalies faster than classical systems. This proactive approach to cybersecurity helps protect sensitive information and maintains the integrity of data systems.
Future Prospects and Ongoing Research
Quantum Machine Learning (QML) started in the early ages and still, it holds the rapid pace of research and development ensuring a promising future. Researchers are diligently working to improve quantum algorithms and hardware, tackling significant challenges such as qubit instability, error rates, and scalability. As advancements in quantum technologies continue, QML is anticipated to become more accessible and practical for a wide range of applications, spanning scientific research to commercial enterprises.
Innovation in QML is being driven by substantial investments from academia, industry, and government. These collaborative efforts are crucial for advancing quantum research centres and supporting startups, which are at the forefront of exploring new possibilities. The aim is to fully leverage the potential of quantum computing, covering the way for significant breakthroughs in artificial intelligence, big data analytics, and other complex problem-solving domains. Additionally, public-private partnerships and international collaborations are playing vital roles in building progress in this field.
Moreover, the integration of QML with other emerging technologies, such as artificial intelligence (AI), the Internet of Things (IoT), and blockchain, could catalyse transformative changes across various industries. This convergence could lead to groundbreaking advancements in smart systems, autonomous decision-making, and real-time data processing. The future of Quantum Machine Learning holds great promise, poised to revolutionise how we interact with and understand complex data, bring on innovation across numerous sectors, and fundamentally alter the technological landscape.
Active Events
Data Scientist Challenges One Should Avoid
Date: April 08, 2025 | 7:00 PM (IST)
7:00 PM (IST) - 8:10 PM (IST)
2753 people have registered
Transition from Non-Data Science to Data Science Roles
Date: April 10, 2025 | 7:00 PM (IST)
7:00 PM (IST) - 8:10 PM (IST)
2753 people have registered
Bootcamps
Data Science Bootcamp
- Duration:8 weeks
- Start Date:October 5, 2024
Full Stack Software Development Bootcamp
- Duration:8 weeks
- Start Date:October 5, 2024