AI Predictive Modeling: Data-Driven Decisions in Dynamic Markets
In today's fast-paced and ever-changing business landscape, making informed decisions is more critical than ever. AI predictive modelling stands out as a transformative tool that enables businesses to navigate the complexities of dynamic markets. By leveraging historical data and advanced algorithms, AI predictive modelling can forecast future trends, customer behaviours, and market movements, thus providing a competitive edge. This article delves into the significance, methodologies, and applications of AI predictive modelling in dynamic markets
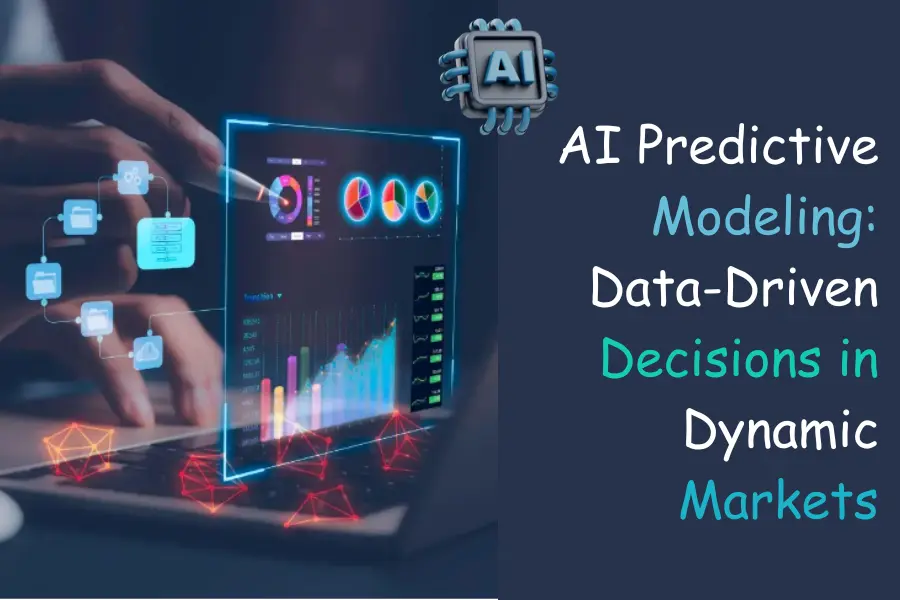
The Importance of AI Predictive Modeling
The primary benefit of AI predictive modelling is its ability to turn vast amounts of data into actionable insights. In dynamic markets, where conditions can change rapidly due to various factors such as economic shifts, technological advancements, and consumer preferences, relying solely on intuition or static data can lead to suboptimal decisions. AI predictive modelling mitigates this risk by offering data-driven predictions that can adapt to new information in real-time.
Predictive models help businesses anticipate trends and prepare for future demands. For example, in the retail sector, predictive analytics can forecast inventory requirements, helping to avoid both overstock and stockouts. In finance, it can predict market trends and guide investment strategies. The healthcare industry uses predictive modelling to forecast patient admissions and improve resource allocation
Methodologies of AI Predictive Modeling
AI predictive modeling employs several sophisticated techniques to analyze data and generate predictions. Some of the most commonly used methodologies include:
- Regression Analysis: This statistical method estimates the relationships among variables. Linear and logistic regression are frequently used to predict continuous outcomes and binary outcomes, respectively.
- Time Series Analysis: This method analyzes time-ordered data points to identify trends, seasonal patterns, and cyclical behaviors. Techniques such as ARIMA (AutoRegressive Integrated Moving Average) are standard in time series forecasting.
- Machine Learning Algorithms: Algorithms such as decision trees, random forests, and neural networks are used to build predictive models. These models can learn from data, identify patterns, and make accurate predictions without being explicitly programmed.
- Natural Language Processing (NLP): For markets where textual data (e.g., news articles, social media posts) influences decisions, NLP techniques help in extracting relevant information and sentiment analysis.
- Ensemble Methods: Combining multiple models to improve prediction accuracy. Techniques like boosting and bagging enhance the robustness of predictions by reducing model variance and bias.
Applications in Dynamic Markets
The applications of AI predictive modeling are vast and varied across different industries. Here are some notable examples:
- Retail and E-commerce: Predictive models analyze customer purchase history, browsing behavior, and external factors such as weather to forecast demand, optimize pricing, and personalize marketing efforts.
- Finance and Banking: Predictive analytics in finance is used for credit scoring, fraud detection, and investment forecasting. Models analyze transaction data, economic indicators, and market sentiments to provide insights.
- Healthcare: Predictive modeling helps in predicting disease outbreaks, patient admissions, and treatment outcomes. By analyzing patient data and medical records, healthcare providers can improve patient care and operational efficiency.
- Manufacturing: Predictive maintenance models analyze sensor data and machine logs to forecast equipment failures, reducing downtime and maintenance costs. This proactive approach ensures higher productivity and efficiency.
- Energy Sector: Predictive models forecast energy demand, optimize power grid management, and predict equipment failures. This helps in maintaining a balance between supply and demand and enhances grid reliability.
Challenges and Considerations
Despite its advantages, implementing AI predictive modelling in dynamic markets comes with challenges. One significant challenge is data quality and availability. Predictive models require large datasets that are accurate, complete, and up-to-date. Ensuring data privacy and security is another critical concern, especially in industries like healthcare and finance.
Moreover, the models must be continuously monitored and updated to maintain their accuracy. Market conditions can change rapidly, rendering older models obsolete. Therefore, businesses need to invest in ongoing model maintenance and improvement.
Active Events
3 Essential Projects to Elevate Your 5c of Content Marketing Portfolio
Date: October 28, 2024 | 7:00 PM(IST)
7:00 PM(IST) - 8:10 PM(IST)
2432 people have registered
Your Data Science Career Game-Changing in 2024: Explore Trends and Opportunities
Date: December 7, 2024 | 7:00 PM (IST)
7:00 PM (IST) - 8:10 PM (IST)
2811 people have registered
Bootcamps
Data Science Bootcamp
- Duration:4 Months
- Start Date:Dec 8, 2024
Data Analyst Bootcamp
- Duration:4 Months
- Start Date:Dec 8, 2024