The Role of AI in Detecting and Preventing Cyber-Attacks in Real-Time
As the digital influence blossoms in strategic management and people’s lives, cybersecurity is the new frontier that one cannot ignore. Due to the higher growth rate of the connected devices and more advanced threats, the need for security mechanisms emerges. Conventional approaches to securing an organisation’s assets are useless as new threats surface, necessitating the development of new techniques. This is where Artificial Intelligence (AI) comes as a solution of a reformation in the way where it is bringing transformation in cyberspace to fight cyber threats in real time.
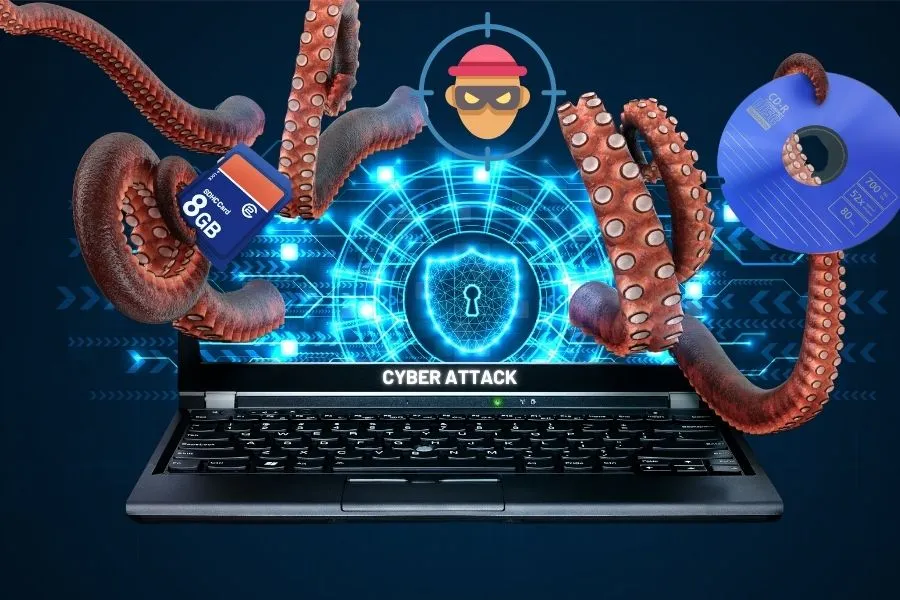
The Evolution of Cyber Threats
New threats have emerged over the years and the threats are becoming more sophisticated and sometimes harder to identify. They are based on predefined rules and signature-based detection and are not well equipped to handle new approaches to attacks. The contemporary threats like zero-day attacks, the advanced persistent threats (APTs), and ransomware evade traditional security solutions.
AI in Cybersecurity: An Overview
AI utilises machine learning (ML), deep learning, and natural language processing (NLP) to process large data sets and extracts signals of the presence of malicious behaviour. Through AI, threat identification and management become automated, which makes it possible for organisations to counter adversaries.
Anomaly Detection
AI is quite useful in the identification of anomalies since a basis for normal behaviour is determined and other behaviours that may represent a cyber-attack are looked at. Real-time monitoring enables machine learning algorithms to scan through the Network traffic, user activities and other activities within a system; anything that seems out of the ordinary calls for an investigation. This is advantageous since it aids in compromising threats that signature-based systems are likely to fail to detect.
Threat Intelligence and Prediction
Specifically, AI systems can combine all the threat intelligence from the forums, social networks, and cybersecurity databases. Through sorting such data, AI is able to establish risk factors and send alerts on these risks. In essence, predictive analytics help organisations enhance strategies to guard against impending attacks, thus minimising the likelihood of a breach.
Automated Response and Mitigation
Once a threat is identified, AI can initiate a response to the threat in a manner that reduces the decision space from threat identification to action. Automated response measures can contain impacted systems and block the traffic that is considered malicious and also trigger handling procedures independently. This is important since a quick response can prevent cyber attack incidents from escalating and causing more losses.
Advanced Threat Hunting
AI plays a role in strengthening the functionality of a cybersecurity analyst by providing him or her with enhanced threat hunting features. Machine learning can extract crucial data without human intervention or in-depth analysis of the situation, in the presence of threats and risks. AI can also be utilised in engineering tools dubbed as the threat hunting tools as these tools can stage a potential symbolic attack and thereby reveal the vulnerabilities of an organisation.
Phishing Detection
Phishing attacks continued as a common form of cyber threats where the attackers sent bogus emails and links to nonexistent websites intending to get details of employees. Machine learning can also be applied to the body of the email, URLs, and sender identification for identification of and prevention of phishing attempts. By applying NLP techs, AI is able to learn about the context and goals of phishing messages and thus enhance the detection process.
Real-World Applications
- Darktrace: Utilising machine learning algorithms, Darktrace's AI platform monitors network traffic and user behaviour to detect anomalies in real-time. Its self-learning technology enables it to adapt to new threats, providing continuous protection.
- Cylance: Cylance uses AI to predict, prevent, and respond to cyber threats. Its AI-driven endpoint protection platform leverages machine learning to identify and block malware before it can execute, reducing the risk of infection.
- IBM Watson for Cyber Security: IBM's AI platform integrates machine learning and NLP to analyse vast amounts of unstructured data, providing actionable insights and enhancing threat detection capabilities.
Challenges and Future Directions
While AI enhances cybersecurity, it faces challenges like adversaries using AI for sophisticated attacks and dependency on high-quality training data. Addressing biases and ensuring comprehensive datasets are crucial. Future advancements in quantum computing, federated learning, and explainable AI will boost AI-driven security solutions. As AI evolves, it will be pivotal in protecting digital assets and maintaining our interconnected world's integrity.
Active Events
3 Essential Projects to Elevate Your 5c of Content Marketing Portfolio
Date: Feburary 25, 2025 | 7:00 PM(IST)
7:00 PM(IST) - 8:10 PM(IST)
2432 people have registered
Unlock Your Career Potential: The 2024 Full Stack Development Success Formula
Date: Feburary 28, 2025 | 7:00 PM(IST)
7:00 PM(IST) - 8:10 PM(IST)
2749 people have registered
Bootcamps
Data Science Bootcamp
- Duration:4 Months
- Start Date:Feb 9, 2025
Full Stack Software Development Bootcamp
- Duration:4 Months
- Start Date:Feb 9, 2025