Clinical Text Mining and Natural Language Processing (NLP)
Clinical text mining has been found to be a revolutionising approach in the healthcare sector in that it makes it possible to extract useful information from large volumes of unstructured clinical texts. These techniques use computational algorithms for text mining of data from a variety of systems such as Electronic Health Records, clinical notes, research articles, etc. to enhance the efficiency of patient care, research, and organisational performance.
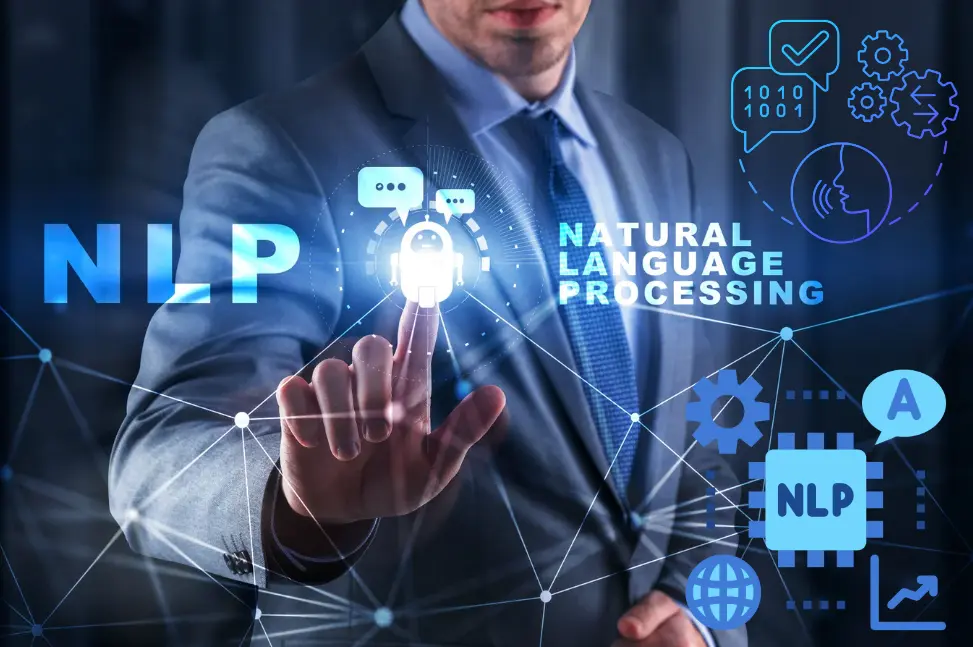
Importance of Clinical Text Mining and NLP
Clinical data may be highly structured, like diagnosis and treatment codes and prescriptions, or loosely structured, like physician notes, discharge summaries, and medical reports. This data is often unstructured, but it holds large amounts of information, and when properly processed and analysed, much can be learned about patterns and system functioning to aid decision making and various applications in the field of healthcare.
Enhanced Patient Care:
Accurate Diagnosis and Treatment: NLP can extract relevant information about symptoms, diagnoses, and treatments from clinical notes, aiding in accurate diagnosis and personalised treatment plans.
Early Detection of Diseases: By analysing patient records and identifying patterns, NLP can help in the early detection of diseases, potentially improving patient outcomes.
Operational Efficiency:
Clinical Documentation Improvement: Automated text mining can streamline clinical documentation, reducing the administrative burden on healthcare providers and improving the accuracy of patient records.
Resource Allocation: Insights from clinical text can inform better resource allocation, such as optimising staffing and equipment usage based on identified needs.
Research and Development:
Clinical Trials: NLP can be used to identify suitable candidates for clinical trials by matching patient records with trial criteria, accelerating the recruitment process.
Medical Research: Mining clinical text helps in identifying trends, associations, and novel insights that can drive medical research and innovation.
Key Techniques in Clinical Text Mining and NLP
Natural Language Understanding (NLU):
Named Entity Recognition (NER): Identifies and classifies key entities such as patient names, medications, diseases, and procedures within the text.
Concept Extraction: Extracts medical concepts and terms from clinical documents, mapping them to standardised vocabularies like ICD-10, SNOMED CT, and UMLS.
Text Classification:
Sentiment Analysis: Determines the sentiment expressed in clinical notes, which can be useful in understanding patient satisfaction and clinician perspectives.
Categorization: Classifies text into predefined categories, such as diagnosis, treatment, or medication, aiding in structured data analysis.
Information Retrieval:
Querying Clinical Text: Enables healthcare professionals to retrieve relevant information from vast amounts of unstructured text based on specific queries.
Document Summarization: Produces concise summaries of lengthy clinical documents, allowing for quick access to critical information.
Relation Extraction:
Identifying Relationships: Extracts and understands relationships between different entities in the text, such as the relationship between symptoms and diagnoses or medications and side effects.
Challenges and Solutions
Data Privacy and Security:
Challenge: Protecting patient privacy while processing sensitive clinical data.
Solution: Implementing robust de-identification techniques and adhering to regulatory standards such as HIPAA to ensure data privacy and security.
Clinical Text Complexity:
Challenge: Handling the complexity and variability of medical language, including jargon, abbreviations, and synonyms.
Solution: Using advanced NLP models trained on large, domain-specific datasets to accurately interpret and process clinical language.
Integration with EHR Systems:
Challenge: Integrating NLP tools with existing EHR systems without disrupting workflows.
Solution: Developing interoperable solutions that seamlessly integrate with EHR systems and support real-time data processing.
Active Events
3 Essential Projects to Elevate Your 5c of Content Marketing Portfolio
Date: April 29, 2025 | 7:00 PM(IST)
7:00 PM(IST) - 8:10 PM(IST)
2432 people have registered
Tips and Tricks for Acing the Data Analyst Job Interview
Date: May 2, 2025 | 7:00 pm
7:00 pm - 8:00 pm
2437 people registered
Bootcamps
Digital Marketing Bootcamp
- Duration:4 Months
- Start Date:May 10, 2025
Data Science Bootcamp
- Duration:4 Months
- Start Date:May 10, 2025